In today’s rapidly evolving technological landscape, machine learning has emerged as a pivotal force driving innovation across industries. From healthcare to finance, and from retail to entertainment, the applications of machine learning are vast and transformative. Understanding how to harness its power effectively can spell the difference between staying ahead of the competition and falling behind in the digital age.
What is Machine Learning?
Machine learning is a branch of artificial intelligence (AI) that empowers systems to learn from data and improve their performance over time without explicit programming. It relies on algorithms that iteratively learn from data, enabling computers to find hidden insights without being explicitly programmed for each task.
Applications Across Industries
- Healthcare: Machine learning algorithms analyze medical data to assist in diagnosing diseases, predicting patient outcomes, and personalizing treatment plans.
- Finance: In finance, machine learning models forecast market trends, detect fraud, and optimize trading strategies based on vast datasets.
- Retail: E-commerce platforms use machine learning to enhance customer experience through personalized recommendations, demand forecasting, and inventory management.
Key Benefits of Machine Learning
- Enhanced Efficiency: Automation of repetitive tasks allows employees to focus on high-value activities, increasing overall productivity.
- Improved Accuracy: Algorithms can process vast amounts of data quickly and identify patterns that humans might miss, leading to more accurate predictions and decisions.
- Cost Savings: By automating processes and reducing errors, businesses can achieve significant cost savings over time.
Challenges and Considerations
While the potential benefits of machine learning are substantial, there are challenges to consider:
- Data Quality: Machine learning models heavily depend on the quality and quantity of data. Poor data quality can lead to inaccurate predictions.
- Algorithm Selection: Choosing the right algorithm for a specific task is crucial. Different algorithms have varying strengths and weaknesses, and selecting the wrong one can impact performance.
- Ethical Implications: Issues such as bias in data and algorithmic transparency require careful consideration to ensure fairness and accountability.
Future Trends
The future of machine learning promises even greater advancements:
- Deep Learning: Subfield of machine learning focusing on neural networks with multiple layers, enabling complex pattern recognition.
- Edge Computing: Processing data locally on devices (e.g., smartphones, IoT devices) rather than in centralized data centers, enhancing real-time responsiveness.
- AI Explainability: Efforts to make machine learning models more interpretable and transparent to build trust and facilitate regulatory compliance.
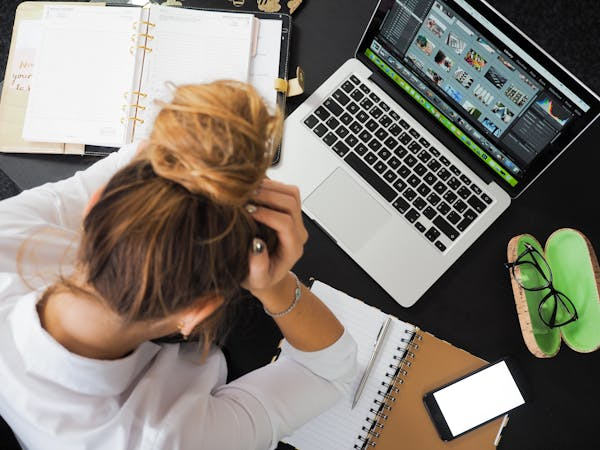
Conclusion
As businesses continue to embrace machine learning, understanding its capabilities and challenges is crucial. By leveraging its power to automate tasks, improve accuracy, and drive innovation, organizations can gain a competitive edge in today’s data-driven economy. As we look ahead, ongoing research and development will unlock new possibilities, shaping the future of machine learning and its transformative impact across industries.
In essence, embracing machine learning isn’t just about adopting new technology—it’s about embracing a new way of thinking and operating in the digital era.
Keywords for High CPC (Cost Per Click): machine learning, artificial intelligence, deep learning, data analytics, predictive modeling, AI algorithms, automated decision-making, business intelligence, machine learning applications